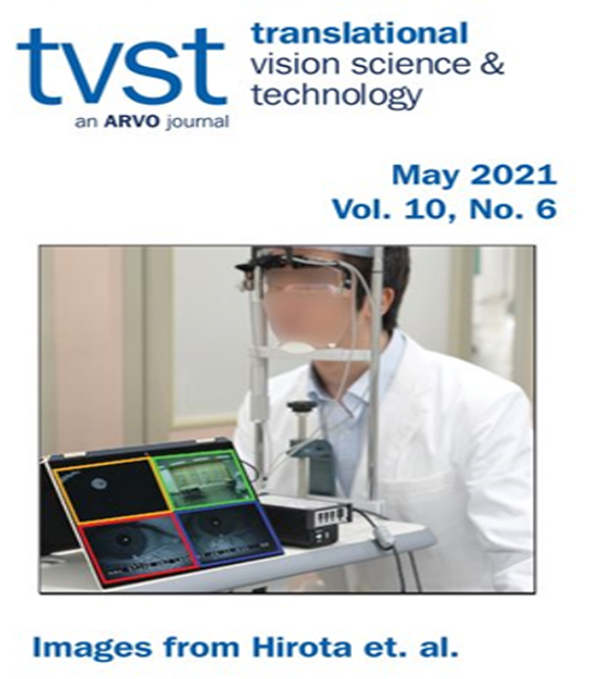
2021
Development of a Web-Based Ensemble Machine Learning Application to Select the Optimal Size of Posterior Chamber Phakic Intraocular Lens
Abstract
Purpose:
Selecting the optimal lens size by predicting the postoperative vault can reduce complications after implantation of an implantable collamer lens with a central-hole (ICL with KS-aquaport). We built a web-based machine learning application that incorporated clinical measurements to predict the postoperative ICL vault and select the optimal ICL size.
Methods:
We applied the stacking ensemble technique based on eXtreme Gradient Boosting (XGBoost) and a light gradient boosting machine to pre-operative ocular data from two eye centers to predict the postoperative vault. We assigned the Korean patient data to a training (N = 2756 eyes) and internal validation (N = 693 eyes) datasets (prospective validation). Japanese patient data (N = 290 eyes) were used as an independent external dataset from different centers to validate the model.
Results:
We developed an ensemble model that showed statistically better performance with a lower mean absolute error for ICL vault prediction (106.88 µm and 143.69 µm in the internal and external validation, respectively) than the other machine learning techniques and the classic ICL sizing methods did when applied to both validation datasets. Considering the lens size selection accuracy, our proposed method showed the best performance for both reference datasets (75.9% and 67.4% in the internal and external validation, respectively).
Conclusions:
Applying the ensemble approach to a large dataset of patients who underwent ICL implantation resulted in a more accurate prediction of vault size and selection of the optimal ICL size.
Translational Relevance:
We developed a web-based application for ICL sizing to facilitate the use of machine learning calculators for clinicians
링크 연결 : https://tvst.arvojournals.org/article.aspx?articleid=2772569
출처 : Translational Vision Science & Technology