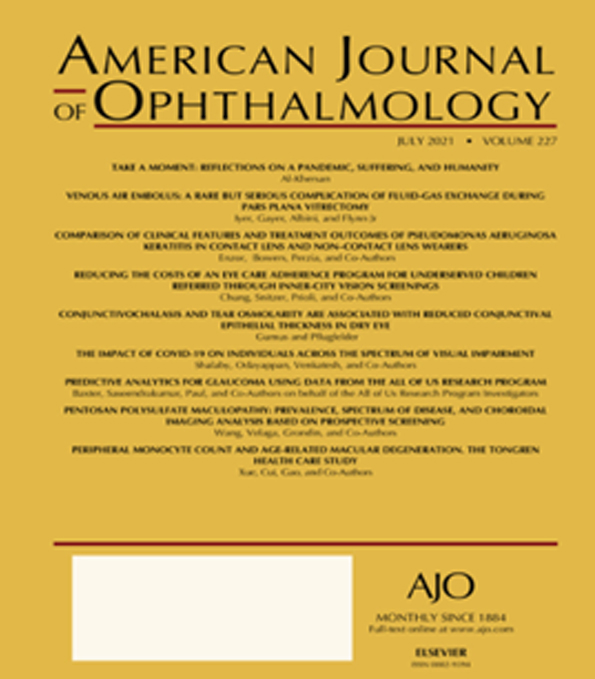
2021
Prediction of Phakic Intraocular Lens Vault Using Machine Learning of Anterior Segment Optical Coherence Tomography Metrics: Phakic lens vault prediction using machine learning
Abstract
Purpose:
compare the achieved vault using the conventional manufacturer's nomogram and the predicted vault using machine learning, in a large cohort of eyes undergoing posterior chamber phakic intraocular lens (EVO implantable collamer lens [ICL]; STAAR Surgical) implantation.
Design:
Reliability and validity assessment and comparison.
Methods:
Our study comprised a total of 1,745 eyes of 1,745 consecutive patients (mean age ± standard deviation, 26.2 ± 6.8 years) undergoing ICL implantation. At 1 month postoperatively, we quantitatively measured the actual vault, and compared it with the predicted vault using machine leaning of anterior segment optical coherence tomography metrics.
Results:
All machine learning methods, such as support vector regressor (SVR), gradient boost regressor (GBR), random forest regressor (RFR), and linear regressor (LR), showed significantly less mean absolute error and higher percentages of eyes within 50-200 µm of the targeted ICL vault than the conventional nomogram (P < .001). The RFR provided the lowest mean absolute errors and the highest percentages of eyes within 50 to 200 µm, followed by the GBR, the LR, and the SVR (P < .01).
Conclusions:
Machine learning of the preoperative anterior segment optical coherence tomography metrics, especially the RFR, provided significantly higher predictability of the ICL vault than the conventional manufacturer's nomogram, suggesting that it will become an aid for predicting the ICL vault and subsequently selecting the proper ICL size in daily practice.
링크 연결 : https://www.sciencedirect.com/science/article/abs/pii/S0002939421000684
출처 : American Journal of Ophthalmology